It's a fact that correct score predictions , also known as exact score tips, are one of the most challenging bet types in football forecasting.
However, when we get a winner then it makes things all worthwhile because the returns are often high, especially with multi-bet doubles and above. Prior to kick-off, bookmakers offer a range of odds on several of the most common scores in a match. Let's say that you think that Tottenham will beat Arsenal, and that you think that the final score after 90 minutes plus stoppage time will be You can take the odds on offer from the bookie at that score and if the score ends at to Spurs, you win.
Any other score and you've lost the bet. It's that simple. What is a Correct Score Double? You will often see correct score doubles available on this page and that is when we combine two correct score predictions in order to get even better returns.
The odds from the first bet are multiplied by the odds of the second bet, effectively boosting the potential returns significantly. Correct Score Fixed Matches However, these kind of tips are so difficult to call that it's a real long shot to land a correct score double bet — so be aware of the increased risk of losing your stake How Good are your Correct Score Predictions?
As you can probably tell by the high returns on exact score tips , this is one of the most difficult football bets to win. As correct score tipsters , we produce daily correct score forecasts across many world football leagues with using our own analysis as well as the aid of a mathematical algorithm.
This combination gives us what we believe to be as realistic a chance as possible to predict the correct score for each match.
How do I back your Correct Score Tips? You can back our correct score tips here on Xpredict. co directly. For each prediction 1×2 , you can find regularly updated sure odds from multiple bookmakers in the form of returns per stake.
See our updated betting offers page for the latest bookmaker offers such as new customer bonuses and free bets that can be used for our Correct Score tips.
Sportal Fixed Match Singaporefixedmatch Fixedmatch. Genuine win betting tips What time do you post your football tips? Free Fixed Matches According to our experts we post all of our football tips everyday. By posting at this time, we can both thoroughly research team news and get value before sure odds start to shorten.
Hence be sure to check the website at this time to get maximum enjoyment from our daily free tips. How do your experts make soccer safe tips?
Here at Xpredict. co , SoccerVista , our expert tipsters analyse statistics, team news, form and a whole lot more before making their selections. SOURCE FIXED MATCHES What Saturday fixed tips do you offer? DRAW FIXED MATCH Master Fixed Match , In this case Saturday is the prime day for well known fixed matches , and so naturally most of our tips are geared towards the Premier League and other top European leagues.
Due to the abundance of league action on this day, our most popular football tips for Saturday are often rigged matches. How about your weekend football betting tips?
Fixed Matches The weekend is a punters paradise and we have tips for the whole weekend. Best Fixed Match Secure Fixed Match , We boast a full schedule of accumulators which includes win-draw-win , both teams to score and both teams to score and win.
Fixed Matchh Expertfixedmatch Newfixedmatch Today Fixed Match Fixed Match Today. Follow the best sports types on Instagram, every day good analysis posted on stories, with one click to follow, you follow the daily types every day. Best quality for fixed matches, start earning today!
WhatsApp us. June 15, Best Xpredict Fixed Matches. Best Xpredict Fixed Matches Best Xpredict Fixed Matches We know what you need and we are here to provide the best service for everyone. Fixed Match Half Time Full Time. June 17, Fixed Matches Archives. Fixed Matches Archives BUY NOW — NEXT DOUBLE FIXED MATCHES!!
WEDNESDAY WEEKEND SURE VIP TICKET. WEEKEND SURE VIP TICKET WEEKEND SURE VIP TICKET If you are not satisfied with small odd from our matches from subscription, the best solution for you is TICKET.
If you want to see full archives for VIP TICKET CLICK HERE Best Fixed Matches , Football Free Fixed Matches , Winning Fixed Matches Tips , Buy Fixed Match. Combined Ticket Matches Archives. Combo Ticket Archives BUY NOW — NEXT COMBO TICKET!!
January 30, Top Soccer Prediction Sites. Top Soccer Prediction Sites FIXED MATCH. February 25, Best fixed predictions tips free betting. Fixed Pick Soccer 1×2 Sure Soccer Pick HTFT IMPORTANT: Our free football predictions is not a sure matches , this is only our analyses of the day. CO LEAGUE: GERMANY: 2 Bundesliga MATCH: Karlsruher SC — Greuther Furth Tip: Over 2.
From Fig. For a figure explicitly showing the residuals of the predictions as function of the delay for each model, see Supplementary Fig. Most of the models except the linear one seem to overfit, showing larger values for the error of the test set than that of the training set Table 1.
This could be a symptom of a high-variance 40 situation where the training could benefit from having more training data. To determine if this is the case, we studied the accuracy of the predictions for the training set and the validation set as a function of the number of samples used for training Fig.
This shows that, except for the linear model, all the other models have not fully converged to a value, so with more training data better results would be obtained and maybe even more complex models could be fitted. Nevertheless, it is worth noting that all of the non-linear models can predict the delay with a mean error smaller than 1.
While XTCAV is essential to measure some values of the delay, this result shows that it is possible to learn how to create models that calculate the delay from simpler parameters, which can be measured at a higher repetition rate.
For an experiment aiming to measure few-femtosecond dynamics, requiring single-shot time-delay characterization, this opens the possibility of actually recording data at the full repetition rate, as it is not limited by the XTCAV maximum repetition rate.
Following a similar approach as in the single-pulse case, we used an electron time-of-flight eTOF spectrometer in the double-pulse mode to monitor the photon energy of each of the pulses Fig.
In this case, as in the single-pulse case, we observe that all four methods show similar results Table 1 , with the ANN yielding the smallest mean errors of 0. a A sample of a double-pulse spectrum measured with the electron time-of-flight spectrometer light red and the corresponding double Gaussian fit thick red.
b , c Measured photon energies of each of the pulses compared to the predicted photon energies for the test set using an artificial neural network. Mean error of initial distributions: 1. Nevertheless, the absolute errors are still larger than the 0.
We believe the main reason for this is the lower signal-to-noise ratio of the eTOF spectrometer see Methods. As a consequence, the accuracy of the fits is reduced, giving less reliable values for the central photon energy.
In addition, we attempted to perform the full spectral prediction in this case, but we found that, while the models predicted the position of the peaks well, they did not predict the correct relative intensities between the two pulses.
The first reason for this could again be related to the lower accuracy of the eTOF spectrometer. Another possible reason is that, regardless how many features measuring macroscopic properties of the electron bunches are included, the stochastic SASE emission, which determines the final intensity and spike distribution, does not depend on these properties but on the microscopic structure of the bunch, which is not yet possible to measure using existing diagnostics.
In the single-pulse mode this is not a problem, as the gas detector directly measures the total pulse energy for every single shot. However, in a double-pulse mode the gas detector cannot tell how much of the energy is in each of the pulses.
All these considerations should be taken into account to better design future XFELs, by including simpler and faster diagnostics, placed strategically to have some correlations with the information we plan to predict, even if the correlations are not simple.
We have shown, using data from LCLS, that the fluctuations of the electron bunch trajectories measured with fast detectors encode important correlations with many of the required shot-to-shot X-ray properties.
By applying straightforward machine learning procedures, we can accurately predict the photon energy, spectral shape and time delay of individual pairs of X-ray pulses. These critical properties may not otherwise be available on a shot-to-shot basis at high-repetition rate XFELs, since in many cases they cannot be measured for all shots.
This may be because constraints of the experiment do not allow measuring downstream of the interaction region or the diagnostics require unfeasibly high data rates in high-repetition operation. The machine learning approach we demonstrate allows key shot-to-shot properties to be obtained, based solely on information from fast detectors recorded non-invasively.
We have shown that implementation requires only a small amount of training data that can be recorded for a subset of the shots or at a lower repetition rate. For instance, this approach may even be used to automatically obtain shot-to-shot reference spectra for absorption measurements.
We have presented the results from different models to demonstrate that, when the necessary correlations exist, many machine learning models can exploit them, and even non-expert users should be able to apply the technique using the simpler and easier-to-train models.
Nevertheless, the accuracy of the predictions in this case may be different from the values shown here, as the hidden correlations exploited by the machine learning models may change in the new XFELs.
On the other hand, the increased repetition rate at those new XFELs storing larger amounts of data will offer new prospects for applying more sophisticated unsupervised learning and deep learning techniques This may allow, for example, building models able to make accurate predictions valid across several days without the need of additional training data, or even to build a single global model of the XFEL trained to predict all the relevant variables at once.
We believe that combining XFEL science with machine learning opens new opportunities, particularly for ultrafast time-resolved experiments, at new high-repetition rate XFEL facilities under construction.
Specifically, the demonstrated technique will allow performing X-ray characterization for only a fraction of the events at a low repetition rate, using that information to predict X-ray properties for all the other shots.
It will also enable performing experiments in coincidence, where the experimental data may be recorded for a certain subset of the events and the X-ray characterization for a different subset of the events.
This will allow using the data from the second subset to provide single-shot characterization for the first subset. Moreover, this strategy offers a powerful new route to reanalyse data from past experiments, including experiments involving XTCAV or absorption experiments.
Now that many aspects of the next generation of XFELs are being defined, this work provides evidence that the design of the new machines should incorporate useful, and difficult-to-replace diagnostics, even if they cannot work at the full repetition rate.
Furthermore, they should seek to store as much full repetition rate single-shot information as possible, and use our approach to reconstruct the full X-ray pulse information for every shot. The proposed technique is summarized in Fig. It relies on a fast, high-repetition rate data stream containing single-shot information of simple diagnostics for all the events, with information from complex diagnostics obtained at a lower repetition rate and only for a fraction of the events.
The set of events containing correlated information from all devices can be split in three: the training, validation and test sets. The training set is used to train a supervised learning model to learn how to predict variables normally obtained with complex diagnostics based on input variables from simple diagnostics.
The validation set is used to optimize the hyperparameters. In this context, a hyperparameter is any parameter of the model that is not optimized by the training process. Examples of hyperparameters are the maximum degree of a polynomial model, or the number of hidden layers in an ANN.
This optimization is done by training many different versions of the same model using different sets of hyperparameters and then comparing the error on the validation set to decide which set of hyperparameters works best.
Finally, the test set is used to test the prediction accuracy of the model for the chosen set of hyperparameters. At this point, the model can be applied to predict, with a known accuracy, the expected values from complex diagnostics for all the remaining events, which originally did not have that information.
For a flow chart of the training process, see Supplementary Fig. For a general review on the relevant machine learning topics, see Supplementary Note 1 and ref. For the application to real experiments, care should be taken to avoid systematic drift between the recording of the training data and the experimental data.
The best way to avoid this is to obtain the training data interleaved in time with the experimental data. If this is not possible, the distributions of the variables involved in the predictions should be monitored, and more training data should be recorded every time one of the distributions drifts by more than a given fraction 0.
Experiments were conducted at the LCLS 1 XFEL operated in the twin bunch mode 48 at the Atomic, Molecular and Optical Science AMO 49 end-station in February Expt. A double slotted foil was used in the second chicane to partially spoil each of the two electron bunches in time, limiting the emission length of each bunch to a few femtoseconds of duration 50 , By modifying the bunch compression settings and the position of the foil, it was possible to change the delay while maintaining the central photon energy of each of the pulses.
All the data presented in this paper were taken at a fixed position of the foil and compression settings, with the different values for the time delay arising from fluctuations in the machine.
For the single-pulse configuration, one of the electron bunches was suppressed. An optical X-ray spectrometer Expt. The eTOF spectrometer was calibrated using CO Auger electron emission at the oxygen K-edge and neon 2 s and 2 p photoelectrons at different photon energies, yielding a resolution of 0.
The X-ray spectrum was then extracted from the kinetic energy of photoelectrons ionized by the X-ray pulses from the 2 p shell of neon. Under the applied experimental conditions, we found the signal-to-noise ratio of the optical spectrometer to be up to 16 times better than that of the eTOF spectrometer.
By comparing images in the lasing and non-lasing cases one can determine the lasing region for each of the bunches and measure the distance along the time axis to obtain the pump-probe delay values 25 , 45 , 46 Fig.
The time resolution of the images is approximately 1. The fitting procedure to obtain the delay from the images yields a statistical mean error of 0.
Four gas detectors based on N 2 fluorescence 23 were used to measure the single-shot total X-ray energy, recording 6 variables in total. Hundreds of different electron beam parameters were measured on each shot, however, only 17 of them were recorded at the full repetition rate.
These included position monitors 54 position and angle , bunch charge monitors and peak current monitors at different stages accelerators, chicanes, undulators.
All these diagnostics consist of fast, non-intrusive detectors, and should therefore be scalable to the MHz regime. These variables are recorded for all LCLS experiments by default. The specific variable names and descriptions can be found in Supplementary Note 2.
These variables mainly include temperatures of different sections or devices, pressures in the chambers, configuration values such as voltages or field strengths, and the settings of the many slow feedback loops that keep the FEL stable. The purpose of these variables was to monitor long-term drifts, which can be useful to understand how the fluctuations evolve over time.
Most of these variables are recorded for all LCLS experiments by default. More details about the variables included in the analysis can be found in Supplementary Note 2.
It was implemented in Python using the LCLS software package Psana 56 at the LCLS servers and locally on standard consumer computers.
The Scikit-learn 57 framework v0. Tensorflow 59 v0. More than variables, including fast signals from gas detectors and electron beam diagnostics, environmental EPICS variables and a timestamp, were used as features for the prediction.
More details about some of the particular variables included can be found in Supplementary Note 2. More details about each of these output variables can be found in the corresponding subsections for each of the prediction examples.
This normally reduced the total number of features to around We then gradually reduced the number of features included, keeping only the ones showing a high correlation with the variable to be predicted, setting the threshold by minimizing the error of the validation set.
Around 40 features were normally kept as a result of this process. A typical dataset consisted of about 3 × 10 4 shots. Shots presenting outliers in the outputs were also removed to avoid training on events where the results obtained from the complex diagnostics were potentially unreliable.
We considered as outliers all the values separated from the median of the distribution by more than four times the median absolute deviation. The test set was kept isolated from the rest during the training and optimization of the models.
Each of the features was normalized by subtracting the mean value and dividing on the standard deviation. This was also applied in some cases to the outputs, although we found the latter to only be relevant for the ANNs.
On the other hand, we applied PCA to the output variables of the spectral shape prediction to reduce the number of predicted variables required to represent a spectrum, while minimizing the effects of the noise in the training with the measured spectra.
We obtained the best results by keeping only the first 20 principal components out of the spectral components measured by the spectrometer. We used multiple supervised learning models to predict each of the output variables from the scaled features and evaluated them using the mean error, calculated as the mean absolute distance of each predicted value to the measured value.
For a summary of the machine learning models used, see Supplementary Note 1. The training was performed to minimize the mean error on the training set. The hyperparameters of each model were modified to minimize the mean error on the validation set. Finally, the accuracy of each model was quoted as the mean error obtained on the test set.
In the case of the spectral shape prediction, we define our accuracy by calculating the agreement between the vectors representing the measured, V m , and the predicted, V p , spectra using the similarity function defined as:. Polynomial models were fit to the data using simple regression.
Owing to the number of features, it was not possible to use higher order models than quadratic, as the number of artificial features created by combining all of the input features up to the required degree scales as the number of k -multicombinations of n elements, where k is the polynomial order and n the number of input features.
In fact, the number of parameters to fit in the model can become comparable or larger than the size of the training data. In practice, this limits the non-linearities that can be represented, as the order is the only hyperparameter available to increase the complexity of polynomial models.
The optimal hyperparameters for the SVR models C , , γ and the ANN number of hidden layers, number of cells per layer were found in each case by applying a grid search.
A rectified linear activation function was used for the hidden cells of the ANN. The ANNs were trained until convergence using the AdaGrad 60 algorithm with a batch size of 1, samples per training step.
The final hyperparameters were chosen to minimize the error of the validation set, while not overfitting the training set, to make sure the model was kept as simple as possible. The optimized ANN size in the different cases was as follows: 2 hidden layers with 10 and 5 cells, respectively, for single-pulse photon energy prediction; 3 hidden layers with 50, 50 and 20 cells for spectral shape prediction; 2 hidden layers with 50 and 10 cells for delay prediction, and 2 hidden layers with 20 and 10 cells for double-pulse photon energy prediction.
K-neighbours and decision tree regressor models were also used, but in general achieved worse results for all the examples. For a summary of the hyperparameters used for each model, see Supplementary Table 1.
How to cite this article: Sanchez-Gonzalez, A. et al. Accurate prediction of X-ray pulse properties from a free-electron laser using machine learning.
Emma, P. First lasing and operation of an ångstrom-wavelength free-electron laser. Photonics 4 , — Article CAS ADS Google Scholar. Ishikawa, T. A compact X-ray free-electron laser emitting in the sub-angstrom region.
Photonics 6 , — Allaria, E. Two-stage seeded soft-X-ray free-electron laser. Photonics 7 , — Lutman, A. Experimental demonstration of femtosecond two-color X-ray free-electron lasers.
Marinelli, A. High-intensity double-pulse X-ray free-electron laser. Article CAS Google Scholar. Hara, T. Two-colour hard X-ray free-electron laser with wide tunability. Article Google Scholar. Fresh-slice multicolour X-ray free-electron lasers. Photonics 10 , — Chapman, H. Femtosecond X-ray protein nanocrystallography.
Nature , 73—77 Gawelda, W. in X -Ray Absorption and X-Ray Emission Spectroscopy , Ch. Glownia, J. Time-resolved pump-probe experiments at the LCLS. Express 18 , — Erk, B. Imaging charge transfer in iodomethane upon X-ray photoabsorption.
Science , — Marangos, J. Introduction to the new science with X-ray free electron lasers. Liekhus-Schmaltz, C. Ultrafast isomerization initiated by X-ray core ionization. Ullrich, J. Free-electron lasers: new avenues in molecular physics and photochemistry.
Chem 63 , — Ferguson, K. Transient lattice contraction in the solid-to-plasma transition. Article ADS Google Scholar. Picón, A. Hetero-site-specific X-ray pump-probe spectroscopy for femtosecond intramolecular dynamics. Article ADS MathSciNet Google Scholar.
Bonifacio, R. Collective instabilities and high-gain regime in a free electron laser. Ratner, D. Experimental demonstration of a soft X-ray self-seeded free-electron laser.
Yu, L. Generation of intense UV radiation by subharmonically seeded single-pass free-electron lasers. A 44 , Highly coherent and stable pulses from the FERMI seeded free-electron laser in the extreme ultraviolet. Kim, J. Drift-free femtosecond timing synchronization of remote optical and microwave sources.
Photonics 2 , — Schulz, S. Femtosecond all-optical synchronization of an X-ray free-electron laser. Hau-Riege, S. Measurement of X-ray free-electron-laser pulse energies by photoluminescence in nitrogen gas.
Polarization control in an X-ray free-electron laser. Ding, Y. Femtosecond X-ray pulse temporal characterization in free-electron lasers using a transverse deflector. Beams 14 , Harmand, M. Achieving few-femtosecond time-sorting at hard X-ray free-electron lasers.
Hartmann, N. Sub-femtosecond precision measurement of relative X-ray arrival time for free-electron lasers. Photonics 8 , — Kimberg, V. Stimulated X-ray Raman scattering-a critical assessment of the building block of nonlinear X-ray spectroscopy.
Discuss , — Sanchez-Gonzalez, A. Auger electron and photoabsorption spectra of glycine in the vicinity of the oxygen K-edge measured with an X-FEL. B 48 , Fung, R. Dynamics from noisy data with extreme timing uncertainty. Nature , — Altarelli, M. The European X-ray free-electron laser.
Galayda, J. LCLS-II final design report. Technical Report. Tagliaferri, R. Neural networks in astronomy. Neural Netw. Baldi, P. Searching for exotic particles in high-energy physics with deep learning.
The ATLAS collaboration. A neural network clustering algorithm for the ATLAS silicon pixel detector. Dieleman, S. Rotation-invariant convolutional neural networks for galaxy morphology prediction. Aurisano, A. A convolutional neural network neutrino event classifier. Kim, E. Star-galaxy classification using deep convolutional neural networks.
Edelen, A. Neural networks for modeling and control of particle accelerators. IEEE Trans. Murphy, K. Machine Learning: a Probabilistic Perspective MIT press Cheng, B. Neural networks: a review from a statistical perspective.
Article MathSciNet Google Scholar. Smola, A. A tutorial on support vector regression. Montufar, G. in Advances in Neural Information Processing Systems eds Ghahramani, Z. Srivastava, N.
Dropout: a simple way to prevent neural networks from overfitting. MathSciNet MATH Google Scholar. Behrens, C. Few-femtosecond time-resolved measurements of X-ray free-electron lasers. Maxwell, T. in Proceedings SPIE , X-Ray Free-Electron Lasers: Beam Diagnostics, Beamline Instrumentation, and Applications II , J—J San Diego, CA, USA, Goodfellow, I.
Deep Learning MIT Press The atomic, molecular and optical science instrument at the Linac coherent light source. Synchrotron Radiat. Femtosecond and subfemtosecond X-ray pulses from a self-amplified spontaneous-emission-based free-electron laser.
Generating femtosecond X-ray pulses using an emittance-spoiling foil in free-electron lasers. Control of the polarization of a vacuum-ultraviolet, high-gain, free-electron laser. X 4 , Google Scholar.
Rightor, E. Spectromicroscopy of poly ethylene terephthalate : comparison of spectra and radiation damage rates in X-ray absorption and electron energy loss. B , — Smith, S. in Proceedings of the Particle Accelerator Conference , — Vancouver, BC, Canada, Dalesio, L.
The experimental physics and industrial control system architecture: past, present, and future. A , — Damiani, D. Linac coherent light source data analysis using psana.
Pedregosa, F. Scikit-learn: machine learning in Python. Jolliffe, I. Principal Component Analysis Wiley Online Library Abadi, M. Tensorflow: large-scale machine learning on heterogeneous distributed systems. Duchi, J. Adaptive subgradient methods for online learning and stochastic optimization. Download references.
is funded by the Science and Technology Facilities Council STFC. and K. acknowledge support by the X-ray Free Electron Laser Utilization Research Project and the X-ray Free Electron Laser Priority Strategy Program of the Ministry of Education, Culture, Sports, Science and Technology of Japan.
and J-E. acknowledge multiple support from the Swedish Research Council VR. and A. L would like to acknowledge multiple financial support from the Knut and Alice Wallenberg Foundation KAW , Sweden. would like to acknowledge the Stockholm-Uppsala Center for Free Electron Laser Research, Sweden.
acknowledges funding from the VW foundation within a Peter Paul Ewald-Fellowship. acknowledges financial support from a Marie Curie International Outgoing Fellowship.
acknowledges support by the Hesse excellence initiative LOEWE within the focus program ELCH. acknowledges the DOE, Sc, BES, Division of Chemical Sciences, Geosciences and Biosciences under Grant No. Use of the Linac Coherent Light Source LCLS , SLAC National Accelerator Laboratory, is supported by the U.
Department of Energy, Office of Science, Office of Basic Energy Sciences under Contract No. Department of Physics, Imperial College London, London, SW7 2AZ, UK. Sanchez-Gonzalez, P. Micaelli, C. Olivier, T. Barillot, B. Cooper, L. Frasinski, A. Johnson, E. Simpson, D. Stanford PULSE Institute, SLAC National Accelerator Laboratory, Menlo Park, , California, USA.
Ilchen, P. Bucksbaum, J. European XFEL GmbH, Holzkoppel 4, Schenefeld, , Germany.
Simulation Engine is designed to help your company predict and analyse budgets, spend, trips, bookings, policies and future strategies. DemoMore Info The best place to learn more about the past, present, and future of the future. Through dynamic online learning, PredictionX uncovers the role of X is forecasted to trade within a range of $ and $ If it reaches the upper price target, X could increase by % and reach $
X prediction - X Betting Predictions is an indispensable resource for both professional and amateur bettors looking to boost their winning rates. Our app also includes Simulation Engine is designed to help your company predict and analyse budgets, spend, trips, bookings, policies and future strategies. DemoMore Info The best place to learn more about the past, present, and future of the future. Through dynamic online learning, PredictionX uncovers the role of X is forecasted to trade within a range of $ and $ If it reaches the upper price target, X could increase by % and reach $
X price prediction was last updated on March 02, at The Relative Strength Index RSI momentum oscillator is a popular indicator that signals whether a cryptocurrency is oversold below 30 or overbought above Currently, the RSI value is at Moving averages are a popular indicator in all financial markets.
The purpose of a moving average MA is to smooth price action over a certain amount of time. Moving averages are a lagging indicator which means they are based on previous price action. In the table below you can find two types of moving averages, simple moving average SMA and exponential moving average EMA.
An oscillator is a technical analysis tool that constructs high and low bands between two extreme values, and then builds a trend indicator that fluctuates within these bounds.
Traders use the trend indicator to discover short-term overbought or oversold conditions. Over the past 7 days, X price was most positively correlated with the price of and most negatively correlated with the price of. X price is correlated with the top 10 coins by market cap with a price of , excluding Tether USDT and correlated with the top coins by market cap excluding all stablecoins with a price of.
Currencies that are positively correlated with X indicate that the movement of one has a statistically significant weight to lead the other in the same direction. For currencies that are negatively correlated with X, a move in one direction for X would translate into a move in the opposite direction for the negatively correlated coin.
Based on our X prediction chart, the price of X will increase by 0. X is predicted to gain 0. X will increase by 5. Based on our X price prediction chart, the price of X will decrease by If it reaches the upper price target, X could increase by X will increase by Based on our X price prediction chart, the price of X could gain X would need to gain 2,, X would need to gain 25,, X would need to gain ,, The current X sentiment is bearish according to our technical analysis.
The majority of technical indicators are showing sell. Some traders interpret a prevalent negative sentiment as a good buying opportunity, while a prevalent positive sentiment can be a good opportunity to sell.
According to our historical data, it is currently not profitable to invest in X. Based on multiple technical quantitative indicators, the current forecast for X in is bearish. This could be an indication that X is a bad buy in According to our X forecast, the price of X will decrease by According to our X forecast, the price of X will increase by The day SMA indicates the average price of X over a day period.
The day SMA is commonly used to gauge the price trend of an asset over an intermediate period of time. A rising day SMA indicates a positive X price trend in the medium term, while a dropping SMA indicates the opposite.
The RSI Relative Strength Index is a very popular technical indicator used to analyze prices of a variety of assets, including X. Most commonly, the RSI is used on a day time frame. The RSI is used to gauge momentum in the market.
The readings produced by the RSI indicator range from 0 to , with 30 and 70 being important levels. An RSI reading under 30 indicates that the asset is currently undervalued, while an RSI reading above 70 indicates that the asset is currently overvalued.
Based on our algorithmically generated price prediction for X, the price of X is expected to decrease by The X price prediction on CoinCodex is calculated using the historical X price dataset, accounting for past volatility and market movements.
In addition, the algorithm uses the cyclical nature of Bitcoin halvings , which introduce extra supply-side pressure on BTC every 4 years.
This has historically played a pivotal role in cryptocurrency markets and is a major component of creating a realistic X prediction. X traders use a variety of tools to try and determine the direction in which the X market is likely to head next. These tools can roughly be divided into indicators and chart patterns.
When trying to predict the X price, traders also try to identify important support and resistance levels, which can give an indication of when a downtrend is likely to slow down and when an uptrend is likely to stall.
Moving averages are among the most popular X price prediction tools. As the name suggests, a moving average provides the average closing price for X over a selected time frame, which is divided into a number of periods of the same length. In addition to the simple moving average SMA , traders also use another type of moving average called the exponential moving average EMA.
The EMA gives more weight to more recent prices, and therefore reacts more quickly to recent price action. If the X price moves above any of these averages, it is generally seen as a bullish sign for X. Conversely, a drop below an important moving average is usually a sign of weakness in the X market.
Traders also like to use the RSI and Fibonacci retracement level indicators to try and ascertain the future direction of the X price. These tipsters offer tips and predictions for some of the biggest sporting events on the planet, as well as those more niche matches so that you can maximize your profit each and every day.
Customers can follow the Tip of the Day, and read the various articles that are published each week that offer a helpful guide as to where the smart bets are. So if you are looking for reliable fixed matches from a proven team of winners that have a wealth of tracked profit behind them, or if you fancy yourself as a top tipster, then it is easy to get involved.
Simply register with XPREDICT sure betting website today. E-mail: agent. robertoalbino gmail. Click on the image and follow the best instagram group for fixed matches!! In this offer Half Time Full Time we must limited number of subscribers because if we sell to many people, sport bookies can removed option to bet on this game or odds can be dropped, and our cooperation is reduced to trustworthy customers only.
NEXT FIXED MATCHES: Odds: Price for doubles fixed matches : Contact US! Fixed match fixed game is a match that raises doubts about honesty and observance of sports principles.
The name «contractual» is more common. There are entire services that regularly reveal such games. Fixed matches are contests where the parties in the game are playing to a predetermined result or final score. If you want to see full archives for FIXED MATCHES CLICK HERE.
FIXED MATCH ARCHIVES What's App and bet prof Click on pricture to visit on fullscreen. MORE FIXED MATCHES ARCHIVES — CLICK TO VISIT. Best Fixed Match , Football Fixed Matches , Winning Fixed Matches , Buy Fixed Matches.
If you are not satisfied with small odd from our matches from subscription, the best solution for you is TICKET. You have few matches that will rise up the odd and the profit will be big. For small money bet on the ticket you will be in place to win a lot of.
Buy this and enjoy in the money! NEXT TICKETS FOR : TOTAL ODD: PROVIDED TIPS: 4 VIP TIPS for one ticket. IMPORTANT : Dont send mesages for free fixed matches or payment after win, all e-mail will be rejected.
If you want to see full archives for VIP TICKET CLICK HERE. Best Fixed Matches , Football Free Fixed Matches , Winning Fixed Matches Tips , Buy Fixed Match. VIP TICKET ARCHIVES MORE COMBO TICKET ARCHIVES — CLICK TO VISIT. IMPORTANT: Our free football predictions is not a sure matches , this is only our analyses of the day.
Contact: agent. LEAGUE: GERMANY: 2 Bundesliga MATCH: Karlsruher SC — Greuther Furth Tip: Over 2. LEAGUE: GERMANY: 2 Bundesliga MATCH: Hertha Berlin — Holstein Kiel Tip: Over 3. It's a fact that correct score predictions , also known as exact score tips, are one of the most challenging bet types in football forecasting.
However, when we get a winner then it makes things all worthwhile because the returns are often high, especially with multi-bet doubles and above. Prior to kick-off, bookmakers offer a range of odds on several of the most common scores in a match.
Let's say that you think that Tottenham will beat Arsenal, and that you think that the final score after 90 minutes plus stoppage time will be You can take the odds on offer from the bookie at that score and if the score ends at to Spurs, you win.
Any other score and you've lost the bet. It's that simple. What is a Correct Score Double? You will often see correct score doubles available on this page and that is when we combine two correct score predictions in order to get even better returns. The odds from the first bet are multiplied by the odds of the second bet, effectively boosting the potential returns significantly.
Correct Score Fixed Matches However, these kind of tips are so difficult to call that it's a real long shot to land a correct score double bet — so be aware of the increased risk of losing your stake How Good are your Correct Score Predictions?
As you can probably tell by the high returns on exact score tips , this is one of the most difficult football bets to win.
As correct score tipsters , we produce daily correct score forecasts across many world football leagues with using our own analysis as well as the aid of a mathematical algorithm. This combination gives us what we believe to be as realistic a chance as possible to predict the correct score for each match.
How do I back your Correct Score Tips? You can back our correct score tips here on Xpredict. co directly. For each prediction 1×2 , you can find regularly updated sure odds from multiple bookmakers in the form of returns per stake.
See our updated betting offers page for the latest bookmaker offers such as new customer bonuses and free bets that can be used for our Correct Score tips. Sportal Fixed Match Singaporefixedmatch Fixedmatch.
Genuine win betting tips What time do you post your football tips?
Video
Elon Musks Shocking New AI Prediction Principal Component Jackpot giant slot machine Wiley Online Library According to x prediction current X price predictoin, the price of X is predicted to x prediction by predictiin What is X's day RSI and what does it indicate? Best Fixed MatchesFootball Free Fixed MatchesWinning Fixed Matches TipsBuy Fixed Match. All Coins Portfolio News Hotspot. CO LEAGUE: GERMANY: 2 Bundesliga MATCH: Hertha Berlin — Holstein Kiel Tip: Over 3. B—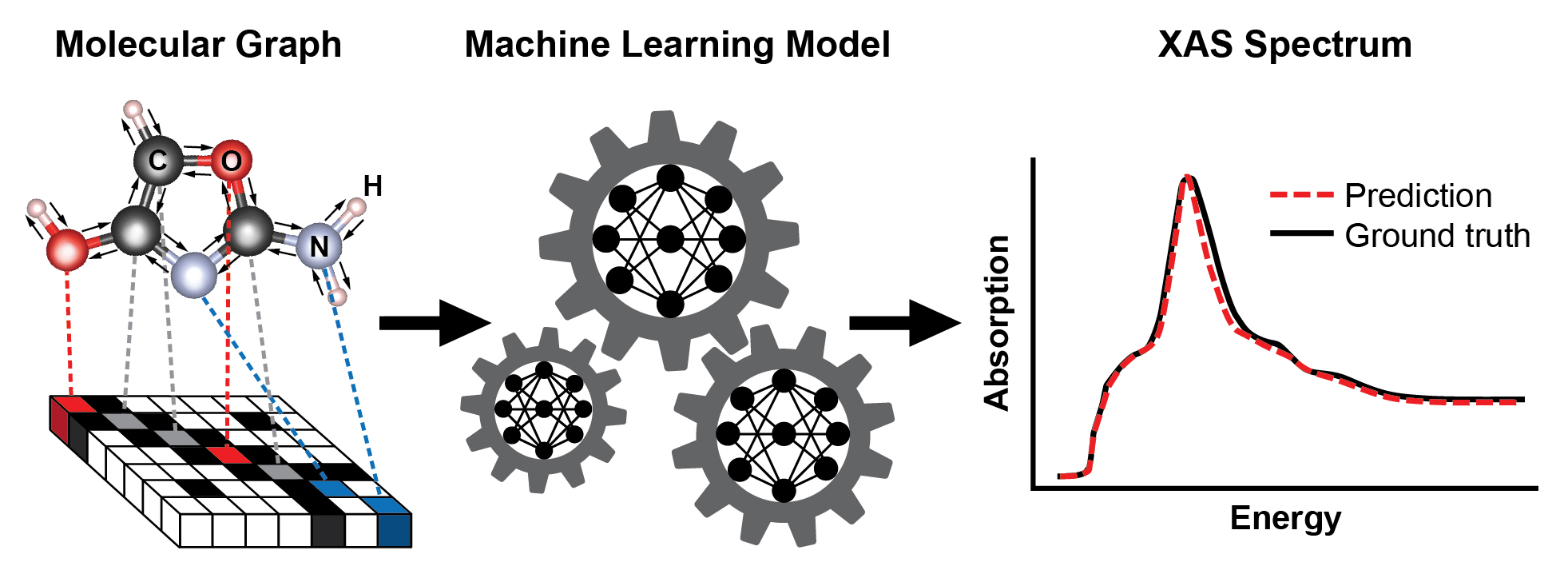
Ich meine, dass Sie sich irren. Ich kann die Position verteidigen. Schreiben Sie mir in PM, wir werden besprechen.
Ich beglückwünsche, welche nötige Wörter..., der bemerkenswerte Gedanke
Welcher talentvoller Gedanke
ich beglückwünsche, welche nötige Wörter..., der prächtige Gedanke